MSc in Health Data Science, skilled in Python and SQL, turning data into strategic insights in healthcare and market research.
Driven by the potential of technology to improve lives, ready for fresh challenges with a mix of analytical prowess and creative problem-solving.
View My LinkedIn Profile
View My GitHub Profile
Objective: The project aimed to apply Time-series Generative Adversarial Networks (TimeGAN) to generate synthetic time-series data based on the CrossCheck dataset for monitoring severe mental illness symptoms. This approach sought to evaluate the effectiveness of synthetic data generation in mental health research, addressing privacy concerns while ensuring data richness and utility.
Methodology:
- Utilised the TimeGAN framework to model and generate time-series data, adapting it specifically for the CrossCheck dataset, which contains granular time-series data on mental health symptoms.
- Conducted data preprocessing to prepare the dataset for TimeGAN, focusing on normalisation and sequence generation to facilitate effective model training.
- Trained the TimeGAN model, fine-tuning its components—Embedder, Generator, Discriminator, and Recovery networks—to accurately capture the data distribution of the CrossCheck dataset.
- Generated synthetic data that mimics the real dataset’s statistical properties and temporal dynamics.
- Employed qualitative and quantitative evaluations, including PCA and t-SNE for visualisation, as well as a novel time series classification approach, to assess the fidelity and utility of the synthetic data compared to the original.
Key Findings:
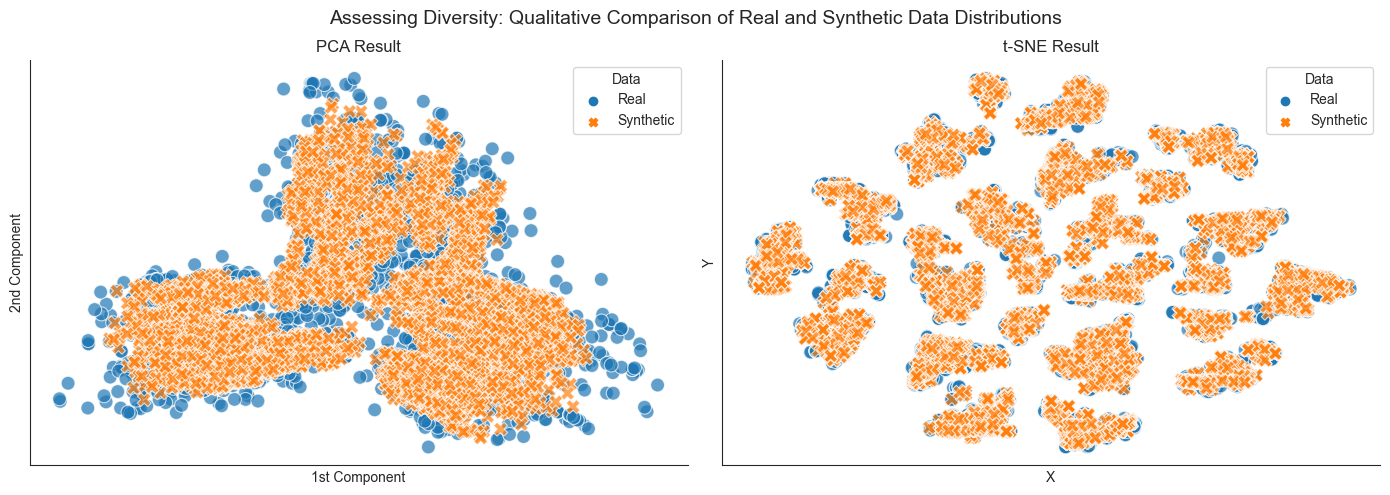
- The generated synthetic data closely resembled the real dataset in terms of statistical properties and temporal patterns, indicating high fidelity.
- Qualitative assessments using PCA and t-SNE visualisations revealed overlapping distributions between real and synthetic datasets, suggesting successful capture of the data’s complexity.
- Quantitative evaluation through time series classification demonstrated that models trained on synthetic data could effectively distinguish between real and synthetic samples, further validating the synthetic data’s quality.
- Comparative analyses indicated that training on synthetic data and testing on real data yielded promising results, underscoring the synthetic data’s utility for research and potential applications in privacy-sensitive contexts.
Implications: This project showcases the potential of GANs in generating high-fidelity, utility-rich synthetic datasets for sensitive applications such as mental health monitoring. By addressing privacy concerns without compromising data utility, this approach opens new avenues for mental health research and the broader field of medical data analysis, where data accessibility is often limited by ethical and privacy constraints.